Multimodel Forecast
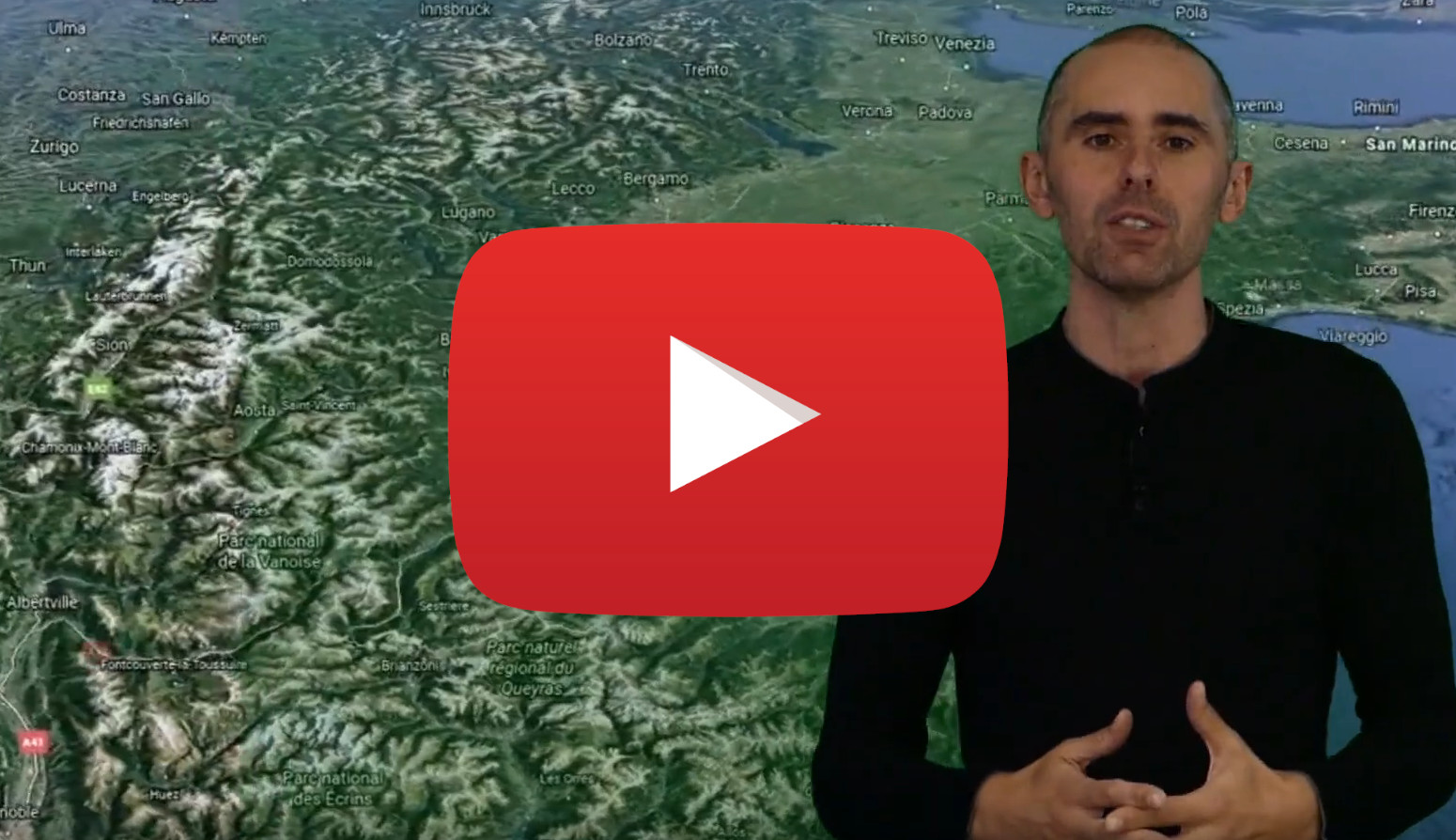
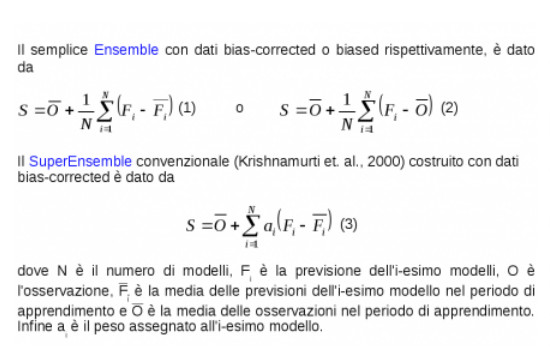
Multimodel SuperEnsemble is a linear autoregressive fit which, using the observed data, determines the weighted average of the various DMO over a period called "training". It is then assumed that this error will be the same over the “forecast” period. In this way, it is possible to obtain a forecast simply by averaging the various available models weighed with this systematic error, different for each one (an error matrix is constructed).
Weights are recalculated every day, for each station and for each forecast time interval. The Multimodel SuperEnsemble forecast is computed every day on the meteorological weather stations of the Italian Civil Protection Network and is able to provide an output even in the absence of one or more models.
A simple Ensemble Forecast (poor man Ensemble) has the following form:
With S the output forecast, the N the Number of input forecasts, Fi the input forecasts, F1 the arithmetic mean of the input forecasts.
An evolution of the poor man ensemble is the bias-corrected Ensemble, that uses this expression:
The Multimodel SuperEnsemble equation is the following, where we introduce the weights ai:
The managed models are the following:
- ECMWF IFS (00 and 12 UTC)
- COSMO-I5 (00 and 12 UTC)
- COSMO-2I (00 and 12 UTC)
- BOLAM (00 UTC)
- MOLOCH (03 UTC)
The (dynamic) learning period is 40 days (it can be varied, if needed). The direct use of the output produced by a global circulation (GCM) or limited area (LAM) meteorological models does not provide optimal results, especially for meteorological parameters close to the surface. This is due to the approximations introduced in the parameterization schemes, the difference between the orography used by the model and the actual orography or an insufficiently accurate representation of the state of the soil. An approach used to provide a more accurate forecast is the development of post-processing schemes, investigating a statistical correction to the direct model outputs (DMO) of these variables.
Since now, many tests have been carried out over the years, with combinations of different models (LAMs alone or LAMs and ECMWF), with different runs (00UTC and / or 12UTC), with different types of "training" periods (fixed or dynamic), with different lengths of the "training" period itself. The results of the tests led to the articulation of the operating procedure with the following characteristics:
- the ECMWF-IFS Global Circulation Model must be included;
- Multimodel forecasts show an added value with respect to the single models output adding up to 8 models;
- dynamic learning allows to consider the seasonal variation of the model's performance, as well as its possible configuration variations